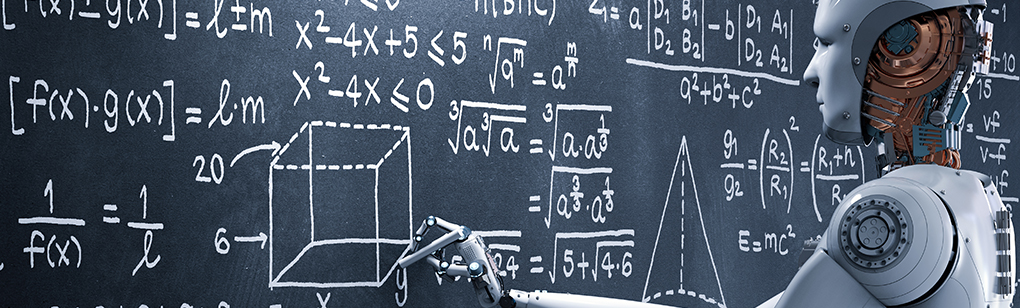
As per ACFE’s 2018 ‘Report to the Nations’, the global fraud loss was estimated to be over 4 trillion. Therefore, it is vital for any bank to monitor, prevent and manage fraud diligently on a continuous basis.
Expectedly, quite a few vendors have come up with Fraud Management offerings, which are primarily rule-based engines. Typically, they monitor transactions of a particular channel and raise alerts / escalations for suspect transactions.
These alerts are then investigated by the bank for investigation and closure. Typically, in a mid-sized bank, there are millions of transactions per day across channels and if the system starts raising alerts even for 10% of those transactions, it would be a huge task for banks to investigate those alerts.
And, imagine 80-90% of such alerts turning out to be false alarms (aka false positives). The bank invariably has to spend time and effort analyzing all these alerts, so as to not allow any potentially fraudulent transactions slip through the cracks.
On the other hand, banks are grappling with the issue of huge false positive rates that is stealing a significant amount of their time and cost. Also, it is de-motivating for the bank’s fraud risk management team to find such an overwhelming number of false positive rates.
In an effort to mitigate fraud, banks shouldn’t end up spending more money than the potential losses due to fraud (imagine spending $1M to investigate a $ 800,000 fraud.!). On the other hand, a typical Fraud Management System cannot be tuned beyond an extent to suppress alerts, as it might mean missing out a genuine fraud.
Banks today are constantly trying to attain a fine balance between these two extremes. Could there be a smarter way to handle the challenge? The short answer is ‘yes’ and here’s where Artificial Intelligence & Machine Learning technology enters.
Let’s take this example. Say the system has a rule that says – ‘whenever the number of transactions per day goes beyond a threshold, raise an alert’.
This threshold could significantly vary from individual to individual. While it could be 2 transactions per day for Mr. Smith, the retired pensioner, it could be a significantly higher number – say 5 for Theo, the young tech-savvy professional who actively uses digital payments including wallets.
Can we make the system intelligent enough to identify who the customer is and accordingly decide if it could be a potentially fraudulent transaction?
Machine Learning can be leveraged to profile customers based on their past transaction patterns and then make an intelligent decision for every subsequent transaction. In this example, if there’s a 3rd transaction for the Mr. Smith on any day, the system should raise an alert (or challenge/decline it) whereas it shouldn’t happen for Theo.
The ability to make this decision dynamically in real-time is the secret sauce which fraud management systems should acquire – and this is very much possible with Machine learning.
Continuing with the example – Based on Theo’s profile, the system finds it is quite fine to allow up to 5 transactions per day. Now, let’s say it is a weekend, Theo is chilling at home browsing Netflix and remembers he has to pay his utility bills before the due date. He takes his mobile phone, pays the heating bill and 2 electricity bills through a wallet, does a recharge of his fiancée’s mobile via the telco’s mobile app.
And, it just so happens that his pre-scheduled insurance policy premium payment date falls due on the same day and it automatically gets processed through his bank’s bill payment ‘auto-pay’. Theo didn’t take any action for his insurance payment, except that he got an SMS informing about this transaction.
After a while, he goes to the neighborhood ATM to withdraw some cash, and whoa! ATM declines his request! His bank suspected this cash withdrawal request to be a possible fraud attempt because there were already 5 transactions since morning and therefore the ATM transaction, the 6th during the day, failed.
The fraud management system deployed in the bank tracked all transactions of the day and was intelligent enough to suspend the next one. So then, is there any issue with the system?
From a system perspective, it learnt Theo’s behavior and acted rightly by rejecting ATM withdrawal, as it is beyond the threshold set for him. From Theo’s perspective, he only paid his routine utility bills which he anyway pays every month and that too they are not big amounts.
Is the system at fault? Can it be made more intelligent? The answer still is ‘yes’. Machine Learning can be further leveraged to handle such scenarios more smartly. Why can’t it learn that the first few transactions are regular bill payments that Theo makes every month and so not flag the next ATM transaction?
Like this, one can continuously fine-tune the system using Machine Learning. Allow your system learn all your customers’ habits, choices, preferences, patterns and then make an intelligent decision.
And who’s happy now? Not just customers like Theo, but the banks also, because they are relieved of investigating such transactions which would eventually turn out to be genuine ones.
So, we see how AI and Machine Learning can be leveraged to address the ‘high false positives’ issue, one of the biggest challenges faced by banks. With smarter systems, both the bank and the customer emerge winners. Of course, the system should make smart decisions not to ignore any potential fraudulent transaction.
While we can keep on tuning the system through Machine Learning, we need to exercise caution in terms of explainability.
For instance, neural network models deployed as part of the Fraud Management System may work more effectively in declining a fraud transaction but it fails to explain why it has declined it. The onus is on the bank to clarify to the customer (or to the audit team) why it stopped that transaction.
The effectiveness of the system should not be at the cost of the ability to explain the reason for a business decision. So, ‘business interpretability’ should be kept in mind while developing advanced models to predict/prevent fraud. This is where the real effectiveness of any system comes into play – it not only takes intelligent decisions, but also has the ability to explain the reason behind a certain decision it took. Otherwise banks would end up with furious customers and unsuccessful audits.
One last thing – while one can leverage Machine Learning as the situation warrants in a smart way, it shouldn’t be force-fit into every situation which could otherwise be handled successfully.
With the advent of newer technologies (like blockchain, for example), we can expect fraudsters to invent more novel attempts. So, it is imperative that the Fraud Management System is continuously enriched to discover emerging fraud patterns and arrest them from occurring.